In today’s edition, we take a look at Google DeepMind’s new efforts to peer inside AI models to dete͏ ͏ ͏ ͏ ͏ ͏ |
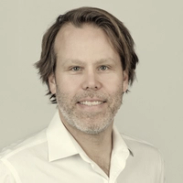 | Reed Albergotti |
|
Hi, and welcome back to Semafor Tech.
Today, we have exclusive news on Google DeepMind’s new framework for evaluating AI models for potentially dangerous capabilities.
This area is going to be heating up, intensifying competition among the big frontier model companies (i.e. Big Tech).
These ideas are so new that it’s difficult to predict how it will unfold, but what we could be seeing is the nascent stages of a global standard for AI evaluation. If companies develop the technology that becomes part of the accepted criteria, they’ll have a competitive advantage. That’s the way standards usually work.
For now, the most powerful AI models are all closed source, meaning the underlying data and “weights” of them are not disclosed.
If “open weights” AI models (like Meta’s Llama family or most models built by Mistral) take over, the evaluation of these offerings could look a lot different.
There are a couple of big takeaways here. First, the companies building cutting edge AI are operating as if this technology is about to get much more powerful and capable. Second, keeping those powerful models in check will be a major undertaking.
This may be what OpenAI CEO Sam Altman was getting at when he said on Lex Fridman’s podcast that most of what OpenAI will eventually be working on is safety. Here’s the full Altman quote:
I think there will come a point where that’s mostly what we think about, the whole company. And it’s not like you have one safety team. It’s like when we shipped GPT-4, that took the whole company thinking about all these different aspects and how they fit together. And I think it’s going to take that. More and more of the company thinks about those issues all the time.
Every step change in AI capability has come after researchers figured out a way to remove some aspect of human involvement. Eventually, much of what goes into making AI more powerful will be discovered by AI itself. At that point, what is left for humans to do?
The answer is probably safety. As I’ve pointed out before, the term “AI safety” has changed in meaning and has become overly broad in a way that may not be helpful.
In this context, what it means is this: Building incredibly powerful AI (if that is even possible) is not the end of the journey. An entire industry is going to be built around ensuring that whatever that AI looks like, it benefits humanity, rather than hurts it.
➚ MOVE FAST: Giving. Reddit is becoming one of the go-to places for AI partnerships. It’s now teaming up with OpenAI to share data. OpenAI will use Reddit’s Data API to obtain text from its chat forums to train ChatGPT, and more, while Reddit will use OpenAI’s AI tools on its own platform to help users and moderators. Timothy A. Clary/AFP via Getty Images➘ BREAK THINGS: Taking. Other publishers, however, are more hostile to AI firms. Sony Music has sent letters to over 700 companies, warning them not to use its audio IP to train and build AI models, including OpenAI, Microsoft, Google, and newer generative AI music startups Udio and Suno, too. |
|
Google DeepMindResearchers criticized Google DeepMind and top science journal Nature for not publishing the code behind AlphaFold 3. Not only does the latest model better predict the structure of proteins, it can also simulate its interactions between other molecules, making it a more powerful tool to design drugs. These capabilities, however, are being kept from scientists, who are unhappy that DeepMind got to publish their research in Nature without having to share its code, going against the journal’s policies. Nature typically requires academics to provide enough details about their research so that it can be validated and reproduced by other experts. But the journal waived this rule for DeepMind. Now, more than 650 scientists have signed a letter to complain that the lack of transparency hampers scientific progress, Science reported. The controversy is a reminder that cutting edge AI research is being developed by private companies that are looking to keep their cards close on how they achieved breakthroughs to commercialize technology. |
|
DeepMind launches framework to assess the dangers of AI |
Samuel de Roman/Getty ImagesTHE SCOOP Preparing for a time when artificial intelligence is so powerful that it can pose a serious, immediate threat to people, Google DeepMind on Friday released a framework for peering inside AI models to determine if they’re approaching dangerous capabilities. DeepMind will work with other companies, academia and lawmakers to improve the framework, according to a statement shared exclusively with Semafor. It plans to start implementing its auditing tools by 2025. Today, evaluating powerful, frontier AI models is more of an ad hoc process that is constantly evolving as researchers develop new techniques. “Red teams” spend weeks or months testing them by trying out different prompts that might bypass safeguards. Then companies implement various techniques, from reinforcement learning to special prompts to corral the models into compliance. That approach works for models today because they aren’t powerful enough to pose much of a threat, but researchers believe a more robust process is needed as models gain capabilities. As that changes, critics worry that by the time people realize the technology has gone too far, it’ll be too late. The Frontier Safety Framework released by DeepMind looks to address that issue. It’s one of several methods announced by major tech companies, including Meta, OpenAI, and Microsoft, to mitigate concerns about AI. “Even though these risks are beyond the reach of present-day models, we hope that implementing and improving the framework will help us prepare to address them,” the company said in the statement. A paper released Friday describes a process in which DeepMind’s models will be reevaluated every time the compute power used to train the model increases six-fold, or is fine-tuned for three months. In the time between evaluations, it says it will design early warning evaluations. Why Reed thinks it’s unclear if the tech exists to make the framework work. → |
|
|
Your daily dose of what’s new in emerging tech. MIT Technology Review’s daily newsletter, The Download, helps you navigate the latest technologies, why they matter, and how they impact the world around us. Sign up for free today. |
|
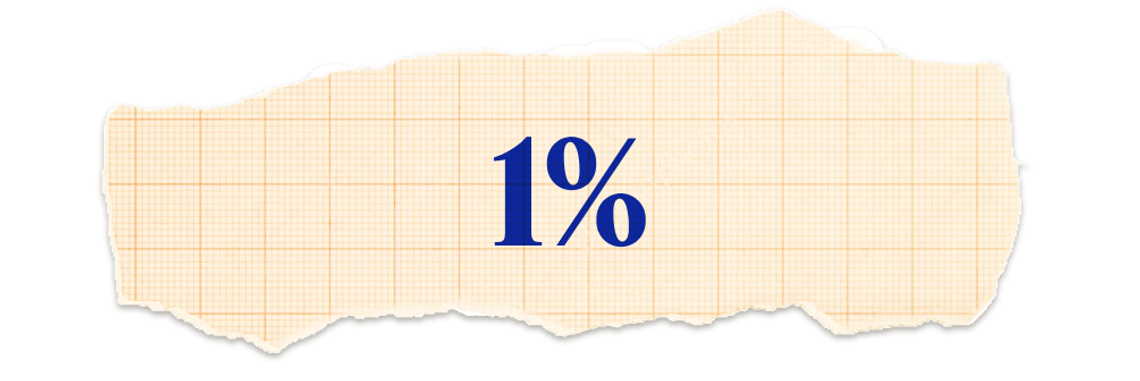 The percentage of Microsoft’s global annual revenue that would be equal to the fine the European Union could impose on the software giant. Microsoft is accused of failing to comply with a request for information on the risks of the company’s generative AI tools. That could cost them billions of dollars. |
|
Arup, a British engineering design consultancy group, was named as the company that lost $25 million in a deepfake scam earlier this year in February, according to the Financial Times. A senior manager was tricked into wiring the money to five bank accounts in Hong Kong in a video call that used AI to digitally clone and mimic the company’s CFO and other employees. The incident is one of the biggest deepfake scams known so far, and the risks will increase as the technology to copy a person’s likeness and voice steadily improves. |
|
Stephen McCarthy/Sportsfile for Web Summit Qatar via Getty ImagesThe AI coding company Replit announced layoffs Thursday, cutting its staff by 25% to about 90 people. I spoke to Replit founder and CEO Amjad Masad yesterday about his tough decision. Just like Tome, which laid off staff last month, Replit is making a move toward enterprise to prioritize paying customers over people who use the product for free. Replit’s customers include ByteDance and Asana, whose engineers use the service to create and test AI-assisted code. Replit launched its enterprise-focused Teams product last month. “We’re going to grow a lot over the next year,” Masad said. “We just need time to do that.” The move by Replit and Tome to cut costs and focus on revenue show how the AI startup landscape is different from the social-media era, where user growth was prioritized over increasing revenue. That’s in part because the AI industry hasn’t yet figured out its money-making business models, so it’s difficult to figure out a value for each user, let alone an expected ARPU (average revenue per user). Add economic uncertainty to the mix and you’ve no doubt got jittery investors who are focusing on cash burn rates at startup board meetings. We’re probably going to see more layoffs in the AI space and even some companies shutting down. And then, eventually, we’ll likely start to see some consumer AI startups take off: The “Ubers and Airbnbs” of the generative AI era. |
|