
The Scoop
The data centers that make generative AI products like ChatGPT possible will soon reach size limits, according to Microsoft Azure Chief Technology Officer Mark Russinovich, necessitating a new method of connecting multiple data centers together for future generations of the technology.
The most advanced AI models today need to be trained inside a single building where tens (and soon hundreds) of thousands of AI processors, such as Nvidia’s H100s, can be connected so they act as one computer.
But as Microsoft and its rivals compete to build the world’s most powerful AI models, several factors, including America’s aging energy grid, will create a de facto cap on the size of a single data center, which soon could consume multiple gigawatts of power, equivalent to hundreds of thousands of homes.
Already, some parts of the national grid are overwhelmed on hot days, when air conditioners are running full blast, forcing rolling blackouts and brownouts.
Microsoft has been working furiously to help add capacity to the grid, inking a deal to reopen the Three Mile Island Nuclear power plant, launching a $30 billion fund for AI infrastructure with BlackRock and inking a $10 billion deal with Brookfield for green energy, among other projects.
Overhauling the US’ energy infrastructure was a big part of the 2022 Inflation Reduction Act, which provided $3 billion in incentives for building out transmission lines, among other priorities. But companies like Microsoft can’t afford to wait around for more money from Washington, on top of the time it would take to deploy those funds.
Microsoft has also innovated on how GPUs are utilized to help data centers run more efficiently.
Given their AI ambitions, a solution could be building data centers in multiple locations to avoid overloading any one region’s power grid. It would be technically challenging, but it may be necessary, Russinovich told Semafor.
“I think it’s inevitable, especially when you get to the kind of scale that these things are getting to,” he said. “In some cases, that might be the only feasible way to train them is to go across data centers, or even across regions,” he said.
Connecting data centers that are already pushing the limits of modern computer networking will be no small feat. Even linking two of them is a challenge, requiring fiber optic speeds that, until recently, were not possible over long distances. For this reason, Russinovich said it is likely the data centers would need to be near each other.
He wasn’t sure exactly when the effort would be required, but it would involve several Microsoft teams as well as OpenAI. It could be years before the effort is necessary. “I don’t think we’re too far away,” he said.
In this article:
Know More
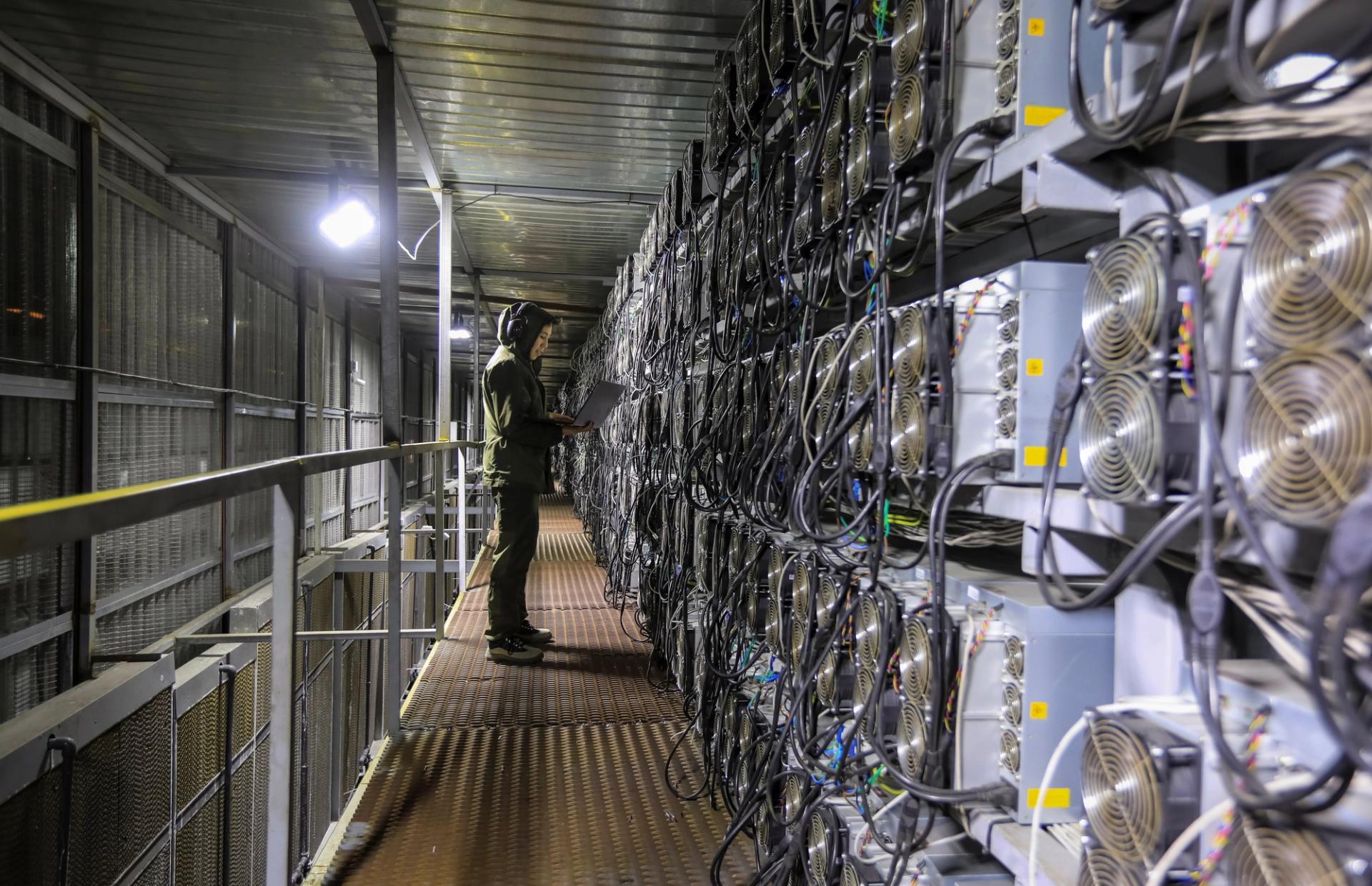
When the largest foundation models are trained, the computation is split up among tens or hundreds of thousands of AI processors (such as Nvidia GPUs). There are many versions of this so-called “parallelization,” but the general idea is to divvy up tasks so that each GPU is working constantly. During the process, data must travel back and forth between all the GPUs.
If you imagined a large foundation model were a skyscraper and construction workers were GPUs, it would be like trying to get them all to work constantly at full speed during the entire project, while simultaneously communicating with one another so that everything got built in the correct order and followed the blueprints.
Creating a perfectly synchronized system to build one massive AI model is a huge technical challenge and it often goes wrong. GPU failures (often from overheating) can ruin a training run.
Even a lag in communication between GPUs could prove disastrous in training. Adding the complexity of multiple data centers spaced out geographically means there are even more things that could go wrong.
Semiconductor analyst Patrick Moorhead, founder and CEO of Moor Insights and Strategy, said data centers are pushing the limits in a lot of areas. For instance, they’ve moved to more efficient liquid cooling systems, something that, before the era of massive AI data centers, was considered unnecessary.
Data centers may reach a point where the cooling systems alone become the bottleneck, drawing too much power from the grid or becoming too inefficient when reaching a certain size.
Chinese hyperscalers have already been experimenting with connecting multiple data centers to train AI models, Moorhead said. Those efforts, however, don’t employ the most powerful AI chips, which are illegal for US companies to sell there.
While connecting two data centers is challenging, some people believe training an AI model might one day be possible with smaller computers spread out all over the world.
Companies like Gensyn are working on new methods of training AI models that can take advantage of essentially any kind of compute, whether from a less powerful CPU or a GPU.
The idea is an exponentially more complicated version of SETI@home, an experiment that allowed anyone to use their computer to help analyze radio telescope data, in hopes of detecting extraterrestrial life.

Reed’s view
For now, training the biggest and most powerful AI models needs to be centralized, even if two or three data centers can train the same model simultaneously.
But if Russinovich’s idea is a first step toward a truly distributed method of training AI models, it would be a big deal and could eventually make AI training more accessible to those who don’t have billions of dollars. It would also mean AI chips, like Nvidia’s GPUs, wouldn’t be as important. You could use less advanced chips but connect more of them to get the same level of compute.
Chip makers are already bending over backwards to make a single processor more powerful. Nvidia’s Blackwell chips are really two separate ones combined. Cerebras makes one the size of a dinner plate. And TSMC is working on ways to make chips even bigger.
What’s cool about the effort to push AI training and inference in the distributed direction is that it opens up new avenues for startups to innovate and potentially disrupt incumbents.