
The Scene
In the AI boom, some startups will win by building the best technology. Others will win by out-executing the competition. One area where execution might matter as much as technology is in customer service software.
Bret Taylor and Clay Bavor, both battle-tested Silicon Valley operators, made a big splash in this space last week when they officially announced their new startup, Sierra, which aims to leverage AI to help companies communicate with their customers.
To take on this new challenge, Taylor left his role as co-CEO of Salesforce and Bavor stepped down as head of Google Labs.
Taylor has also been all over the tech headlines over the last couple of years. He led Twitter’s board of directors through its tumultuous courtship with Elon Musk. And he recently joined OpenAI’s board amid the ouster and rehiring of CEO Sam Altman.
Read below for a wide-ranging, edited conversation on their new startup, OpenAI, and their views on the greater AI ecosystem.

The View From Bret Taylor and Clay Bavor
Q: When you decided you wanted to start a company, did you know what you were going to do?
Bret: We knew we wanted to empower businesses with this technology. One of our principles was, it’s hard, even in San Francisco, to follow the pace of progress. Every day, there’s a new research paper, a new this, a new that. Imagine being a big consumer brand. How do you actually take advantage of this technology?
It’s not like you can read research papers every week as the CEO of Weight Watchers. So we knew we wanted to enable businesses to consume this technology in a push-button way, a solution to bring this to every company in the world. We called on a lot of CIOs, CTOs, and CEOs of companies we worked with in the past. We talked to them about the problems they were facing. Through that, we got excited about one concept, which is the future of digital customer experiences, thinking of this asset, which is the AI agent, as being a really important new concept.
It wasn’t just about customer service; it was something bigger than that. We always talked about people’s websites and apps, that’s their digital identity. In the future, every company will need an agent. ‘Can you update the agent with the new policies?’ That sentence will come from a CEO’s mouth at some point. What we loved about it, when you’re starting a company, you want to imagine yourself doing it for 20-plus years. So it’s a big commitment. We love that there was a short-term demand in customer service where we could improve something very expensive that no one likes much. So it’s a great application of AI.
Clay: One of the things that we’ve been very focused on from the beginning is being intensely customer-led. Back to your question on how we started, it was through a series of conversations rather than taking this new technology and being the hammer trying to find the proverbial nails.
Q: Would you want to develop your own models?
Bret: We converged on a technical approach that we should not be pre-training models. Our area of AI research is around autonomous agents, it’s really thriving in the open-source community. There’s a lot of people making an AI that’s answering all my emails, which is an energetic, open-source community that is fun to watch.
There are a couple of reasons why we really believe in it. One is customer benefits. Imagine it’s Black Friday, Cyber Monday, and you want to extend your return period to past New Year’s, which is a common thing to do. If you’re building your own pre-trained model, you’re like, we can update the policy in like three weeks. And that’s going to cost $100,000. The agility that you get from using a constellation of models, retrieval-augmented generation, all the common techniques in agentic style AI.
Similarly, it means we can serve a much broader range of customers because it’s not like you have to build an expensive model for every customer. And like Reid Hoffman’s characterization, there are frontier models, like GPT-4 and Gemini Ultra. And then there are foundation models, which is just this broad range of open source and others. The foundation models are sort of a commodity now. It makes a lot of sense to focus on fine-tuning and post-training and say, ‘we can start with these great open-source models or other people’s foundation models, and just add value, which is unique to our business.’ For example, we have a model that detects ‘are you giving medical advice?’ We have a model that detects ‘are you hallucinating?’ The pre-training part of that isn’t particularly differentiated.
So our view is that there’s a Moore’s Law level of investment in these foundation models. We’d rather benefit from that rising tide lifting our boat, rather than burning our own capital, doing what is a relatively undifferentiated part of the AI supply chain, and really focus on what makes our platform unique. If you squint, it’s like the cloud market. How many startups build their own data centers now? For some companies, it might make sense, but you should have a very specialized use case. Otherwise, licensing the server from Amazon or Azure probably makes a ton more sense. I think the same is true of these foundation models.
Q: Has the process of building an autonomous agent been more challenging than you thought it would be?
Clay: It’s been incredibly fun exploring this territory because you can anthropomorphize how humans think, reason, and recall things, and those really apply to so many things in developing agents. For instance, what does it take to respond effectively to customers and solve problems? You have to plan. How should the agent go about planning?
So we have specialized models that are experts in planning and thinking through the next steps. How do you answer a factual question about the company? You recall a memory of something you read previously. So we’ve figured out how to give our agent access to, in essence, a reference library that it can read through in an instant, pull out the right bits, and use those to summarize and synthesize an answer. How do we make sure that answers are factual or the action that the agent is taking is correct?
We have another module within our agent architecture that we affectionately call the supervisor. And the supervisor, before a message is sent to a user or an action is taken, will basically review the agent’s work, and say, ‘actually, I think you need to make a little change here, try again and get back to me,’ and only after the initial process has revised that, will the action be taken or the response sent.
On what’s been hard, there are a number of really important challenges that if you’re going to put AI directly in front of your customers, you need to mitigate and overcome. For hallucinations, large language models can synthesize answers and facts that aren’t, in fact, factual. So we built a layered approach to ensuring that we can mitigate hallucinations, and there’s no guarantee because AI is non-deterministic. We’re using supervisory layers, giving it access to knowledge provided by the company. We’re providing audit and inspection tools, and quality assurance tools, so that our customers can review conversations and, in essence, coach the AI in the right direction through this feedback mechanism.
No matter how smart one of these frontier models is, it’s not going to know, Reed, where your order is, or when I bought my shoes and whether or not they’re eligible for returns. So you have to be able to integrate safely, securely, and reliably with the systems that you use to run your business. And we’ve built some really important protections there where all actions taken when you’re interacting with customer or company data are completely deterministic. They use good old-fashioned if-then-else statements, and don’t rely on LLMs, and their unpredictability to manage things like access controls, security, and so on.
The last interesting challenge has been, of course you want an AI agent representing your company to be able to do stuff, to answer questions, to be able to solve problems. But you also want it to be a good ambassador of your brand and of your company. So one of the most interesting challenges has been, how do we imbue a company’s AI agent with its values and its voice, its way of being?
One of our design partners, OluKai, is a Hawaiian-inspired retailer. They wanted to make sure that their AI agent interacts with what they call the Aloha experience. So we’ve imbued it with tone, language, some knowledge of the Hawaiian language. We’ve even had it throw the shaka emoji at a customer who was particularly friendly towards the end of an interaction.
One of our other customers has what they refer to as the language of luxury, a kind of a refined way of interacting with customers with really excellent manners. These are some of the challenges that we’ve had to overcome. They’ve been hard but really interesting.
Q: When people think of automated customer service, the thought is, ‘how do I get to a real person in the quickest way?’ Are you seeing evidence that people might enjoy talking to a robot more than a person?
Bret: That’s definitely our ambition. So Weight Watchers, the AI in their app is handling over 70% of conversations completely autonomously. And it’s a 4.6 out of 5-star customer satisfaction score, which is remarkable. OluKai, over Black Friday, Cyber Monday, we handled over half their cases with a 4.5 out of 5 customer satisfaction score. The joke we all say is if you surveyed anybody, ‘Do you like talking to a customer support chatbot?’, you could not find a person who says ‘yes.’
I think if you survey people about ChatGPT, you get the inverse. Everyone loves it, even with its flaws, and hallucinations. It’s delightful. It’s fun. That’s why it’s so popular. One of our big challenges will be to shift the perception of chatting with an AI. At our company, we don’t use the word bot, because we’ve found that consumers associate it with the old technology.
So our customers get to name their agent, but we usually refer to it as an AI or an agent or a virtual agent, to try to make sure that the brand association is ‘hey, it’s this new thing, it’s this fun, delightful, empathetic thing, not that old, robotic thing.’ But it’ll be an interesting challenge.
Our AI agents are always on, faster, more delightful than having to wait on hold, not because the agent on the other side is bad. But you don’t have to wait on hold. It’s instantaneous. It’s faster. I hope that we end up where people are like ‘ don’t you have an AI I can talk to? Are you kidding me? I have to talk to a real person?’ I don’t think we’re there, and I think there’ll be a bit of a cultural shift. We’ve even talked about how do you actually know you’re talking to one of the good ones versus the old bad ones? Because they kind of look the same. But you know it when you see it.
Q: There are some really heavy hitters in this space trying to do something similar. How do you differentiate yourselves?
Bret: We’re really focused on driving real success with real scaled consumer brands like Sonos, Sirius XM, Weight Watchers, and OluKai. We really recognize that it’s very easy to make a demo in this space, but to get something to work at scale, that’s where the hard stuff is. When companies decide who they want to partner with, they’ll look at who are the customers? Do I respect them?
We want to be focused on the enterprise. We believe that the needs of enterprise consumer brands are pretty distinct or higher scale. They have really strict regulatory requirements that smaller companies don’t have. That produces a platform where we have a lot of enterprise features around protecting personal identifiable information, compliance, things that are an important category of enterprise software that I think will set us apart.
We also have a really great business model. We call it outcome-based pricing. Our customers only pay us when we fully resolve the issue. It means that they are only paying us when we’re saving them money. It will be competitive and execution really matters. The company hasn’t even existed for 11 months and we’ve got live paying customers.
Very few people remember AltaVista, but those of us at Google at the time do. Very few people remember Buy.com; they remember Amazon. We’re aware that in these periods of technology innovation, execution matters a lot.
Q: Just to make sure I understand, if I’m a customer and I go to a human, then that company doesn’t have to pay you because the agent did not resolve the issue..
Bret: That’s right.
Q: You’re a startup. You have no time to be distracted. But then you became chair of the OpenAI board. What was that like for you two then?
Bret: The reason why I agreed to join the board was a sense of the gravity and importance of OpenAI. I had this genuine fear that the OpenAI that had produced so much of the innovation that inspired Clay and I to quit our jobs might cease to exist in its current form. I was in a unique position to help facilitate an outcome where OpenAI could be preserved, and I felt a sense of obligation to do it.
When I talked to Clay, the conversation was like, ‘is this going to take too much time? Is it going to be a distraction?’ Both of us were like, ‘OpenAI is really important.’ You’re not going to sit around 10 years from now and say, ‘was it a bad use of time to help preserve the mission of ensuring Artificial General Intelligence benefits all of humanity.’ I’ve served on public boards before, including some high profile ones. I’ve been pretty good at time management and work a lot. We’ve been able to manage it pretty well. At the end of day, we’re technologists.
It’s funny. Now people ask, ‘is it competitive?’ It’s like asking, ‘is the internet a market?’ I don’t think it is. If I have to articulate the AI market, there’s infrastructure, there’s foundation model providers, there’s tools, and then there’s solutions. We’re a solution. We’re in a different part of the supply chain of AI.
Clay: As we do with everything, we talked it through. And I really felt, and I think Bret felt, that there was an element of civic duty. It’s fair to say that Bret was in a literally unique position to make a difference, given his experience, given his great mind, and perhaps most importantly, given his values and judgment. For the impact on Sierra, Bret has done a remarkable job balancing everything and I’m really proud to, from a step removed, be a part of preserving this really important organization.
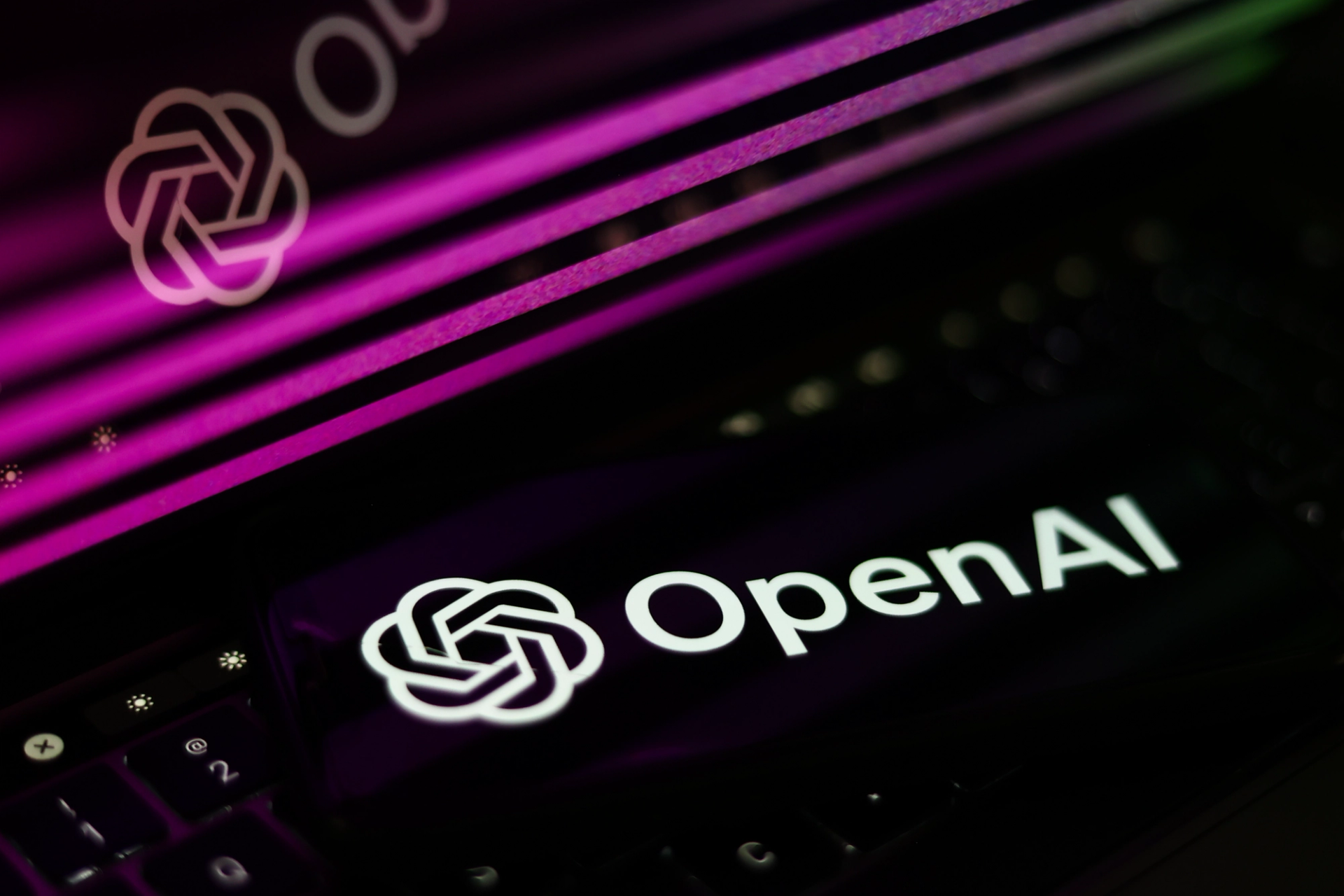
Q: I think every company in crisis now is going to call you to be on their board.
Bret: I’m trying to figure out the reputation I have now. Am I like Harvey Keitel from Pulp Fiction or something? I don’t know.
Q: Is the drama over, by the way? I know there’s an ongoing investigation.
Bret: Nothing to share at this point. But over the coming months, we’ll be super transparent about all of that.
Q: Speaking of AGI, I know you’re not developing it. But has this experience of trying to meld all these different models, and fine-tune them to build something more intelligent, made you think about the path to AGI any differently?
Bret: I’m not an expert in AGI so take this as a slightly outside, slightly inside perspective. I do think that composing different models to produce something greater is a really interesting technique. If you have a model that’s wrong 10% of the time and right 90% of the time, and another model that can detect when it’s wrong with the same level of accuracy, you can compose them and make something that’s right 99% of the time. It’s also slower and more expensive, though, you end up with a pipeline of intelligence. There’s both time and cost limits to it. But it’s really interesting architecturally.
The biggest trend change that Clay and I have talked about is, I think three years ago — ancient history — AI was sort of the domain of machine learning. You meet a data scientist, their workflows are very different than engineers. It’s like notebooks and lots of data. Source control is optional. It’s very different culturally than traditional software engineering. Now, particularly with agent-oriented models, you can use models off the shelf, you can wire them together, and AI has moved to the domain of engineering.
You use it almost like you think of spinning up a database or something like, ‘oh, yeah, we’ll use this model for that and use this model for this.’ I’m not sure of its impact on AGI, which has a lot of connotation, but certainly as it relates to building an intelligence into all the products we use on a daily basis, I think it’s been democratized.
LLMs just enable transfer learning. Essentially, when you train on all of human knowledge, it’s very easy to get it to do something smart at the tail end of that, kind of reductively. As a consequence, that’s so interesting, because now just every day full stack developers can incorporate next generation intelligence into their product. You used to have to be Google.
Now it’s like, everyday programmers have these at their disposal. And I still think we haven’t seen the end of that. The first generation of iPhone apps were like a flashlight. I think the early AI applications were sort of thin wrappers on top of ChatGPT. We haven’t gotten yet to the WhatsApps and the Ubers.
Q: I also wonder if there’s also an element of the early internet here, where there’s an infrastructure bottleneck. You can’t use a frontier model for every part of this. It’s too slow, too expensive. So, do you try to make your software efficient for today’s models, or make it a little inefficient in anticipation of the infrastructure layer improving?
Bret: Our approach internally with research is to use overpowered models to prove out a concept and then specialize afterwards. And I really think that style of development is great. It’s like vertical integration, you can get it working, prove it out, and then say, ‘Okay, can we build specialized models?’ There’s been a lot of research — Microsoft had, I can’t remember the name of the research paper — but there’s been a ton of research of using very large parameter models to make lower parameter calls that are really effective.
Q: Textbooks Are All You Need.
Bret: That was the paper. This area is fascinating. One of the things we’ve talked about is Sierra was the name of a game software company in the 90s that both of us played. I remember hearing stories of the game developers in the ‘90s, where they’d make a game for a computer that didn’t exist yet. Moore’s Law was at such a blistering pace at that point that making a game for the current generation just didn’t make sense, you’d make it for the next one.
When we think about Sierra, we think about two forms of this, which is one you can build with lower parameter, cheaper models that make it faster and cheaper. Similarly, even the current generation of models will be cheaper and faster a year from now, even if you did nothing. So there’s this interesting thing as you’re building a business and you’re thinking about your gross margins, which is talking about the present will be the past so quickly, it’s almost incorrect. You really actually should be thinking about Moore’s Law the way a ‘90s game developer thought about the PC.
It makes it very hard to form a business plan, by the way, because you almost have to bet on the outcome, but you don’t have all the information. We know a multimodal model that supports x is going to exist by the end of this year, with like a 90% likelihood. What decision do you make as a technologist at this point to optimize for that? It’s fun, but it’s chaotic.
Q: It sounds like getting that exactly right might be the thing that makes you win.
Clay: Being able to read the trend lines and how quickly these new capabilities will come from being just over the horizon, to on the horizon, to available and usable for building new products with, that’s part of the art here. We both often fall asleep reading research papers at night. So we’re up to speed on the latest. Our hope is that we can read those research papers and hire the PhDs so that our customers don’t have to, and we can enable every one of them to build this AI agent version of themselves.
Q: You’ve said that this will put some call center workers out of business, but it will also create new jobs. I agree but do you have any ideas of what those new jobs will be?
Bret: One of our design partners, the customer experience team, they’re in the operations part of the customer service team. They were doing quality assurance on the agent, including both before and after launch, reporting issues with live conversations. They refer to themselves now as the AI architects and their main job is actually shaping and changing the behavior of the AI. We’ve embraced that.
With our new customers, we talk about how you need to have some people adopt this AI architect role. The exciting part for me is what is the webmaster of AI? Not the computer science person who’s making the hardcore HTTP server, but the person whose actual job it is to help a company get their stuff up and running, and maintain it.
We love this idea of an AI architect, but I think it requires technology companies to create tools that are accessible to people who are not technologists so that they can be a part of this. I actually was really inspired by the role of Salesforce administrator. It would surprise me if it weren’t one of the top 10 jobs on Indeed still to this day. And the role of a Salesforce administrator is a low code, no code job to set up Salesforce for people.
If you talk to Salesforce administrators, 99% of them made a mid-career transition to that role. Everything from manicurists to accidental admin, like your boss says, ‘hey, we have the Salesforce thing, you mind maintaining it?’ Ten years later, they have a higher salary and they’re part of this ecosystem.
It’s important as technology companies, we’re creating those opportunities to have on-ramps for people from operational roles around service to benefit from the rising tide of all the investment in this space. It will be disruptive, though. I don’t know the history of the automated teller machine very well. I imagine there was a point where it was disruptive. And it’s very easy to say now that bank employees didn’t go down. What about the week you put it in? Was that moment disruptive? It probably was.
We shouldn’t be insensitive to the fact that when you start answering 70% of conversations with an AI, there’s probably a person on the other side that’s getting less traffic. That’s something we need to be accountable to and sensitive to. But the average tenure of a contact center agent is way less than two years. It’s not a career people seek out. It’s not necessarily the most pleasant work. If you see in a call center, people have eight chat windows open at the same time, with a requirement of how many conversations they can have per hour. It’s a challenging job.
So I’m hopeful that the jobs that come out of this will be better and more fulfilling. But the transition could be awkward, and that’s something we need to be sensitive to and it’s something Clay and I talk a ton about.