
The News
Can a machine mimic a human trying to mimic a machine better than a human can?
Short answer: Yes. Longer answer: Yes, and it matters — in particular to a kind of high-stakes, everyday journalism that mixes qualitative and quantitative assessments, and which I suspect is pretty much the last thing most editors would entrust to AI.
Bear with me.
Large Language Models like ChatGPT are often derided — accurately — for the way they embody our many human flaws: inexactness, inconsistency, fuzzy thinking. Shouldn’t machines be able to do better, you ask. But what if those traits could be features, not bugs?
I was mulling these ideas during a class I’m co-teaching on computational journalism (I know, too much free time) and listening to a presentation on how hard it is to accurately collect hate crime statistics. The Department of Justice definition is pretty straightforward, but police departments around the country track them in different ways, often with different criteria. A massive annual DOJ survey of people’s experience of crime yields widely different results from the official numbers. How to square those circles?
One way is by trawling through hundreds of crime stories in local news sites to get a sense of what’s happening on the ground; a tried-and-tested journalism and research technique. There are three common ways to sort out which stories might refer to hate crimes and which might not: Write an incredibly detailed search term for key words in the stories; use some form of machine learning, feeding in hundreds of examples of hate crimes and hundreds that aren’t, and have the machine figure out what distinguishes one from the other; or pay a large number of people a little bit of money each to rate the stories as likely to be hate crimes or not (a process known as using a Mechanical Turk, so named for a fake chess-playing machine in the 1700s that actually had a human in it).
The first system is tough to pull off in practice; how can you be sure you’ve captured every possible nuance of every possible hate crime in your terms? The second is prone to error — who knows what false associations the machine might make, and how many existing biases in the data it will encode? And the third takes advantage of human understanding of nuance and complexity to identify hate crimes, but requires time, money and people.
But what if machines could substitute for people?
That’s what I set out to do.
In this article:
Know More
It’s not hard to build a Mechanical Turk bot. I took the DOJ definition of a hate crime and put it into a GPT4-powered bot, told it not to use any other information, and asked it to assess (made-up) news stories on how likely it was they referred to a hate crime. And then I fed a steady diet of those stories.
The first described an attack and robbery of a woman in Brooklyn by a group of five assailants, captured on video. Neither the victim nor the attackers were identified, and the bot scored the likelihood of this being a hate crime as “very low.”
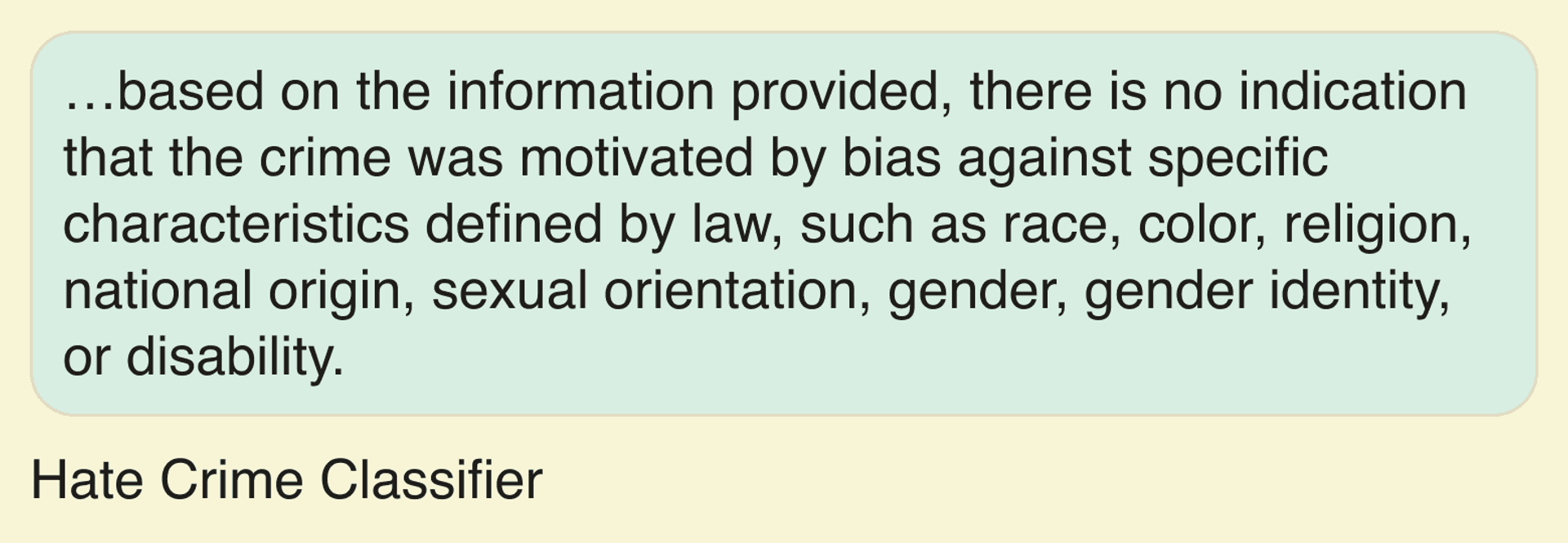
I modified the story to describe the victim as an Asian woman, and added the detail that one of the attackers was heard saying “we don’t want your kind here.”
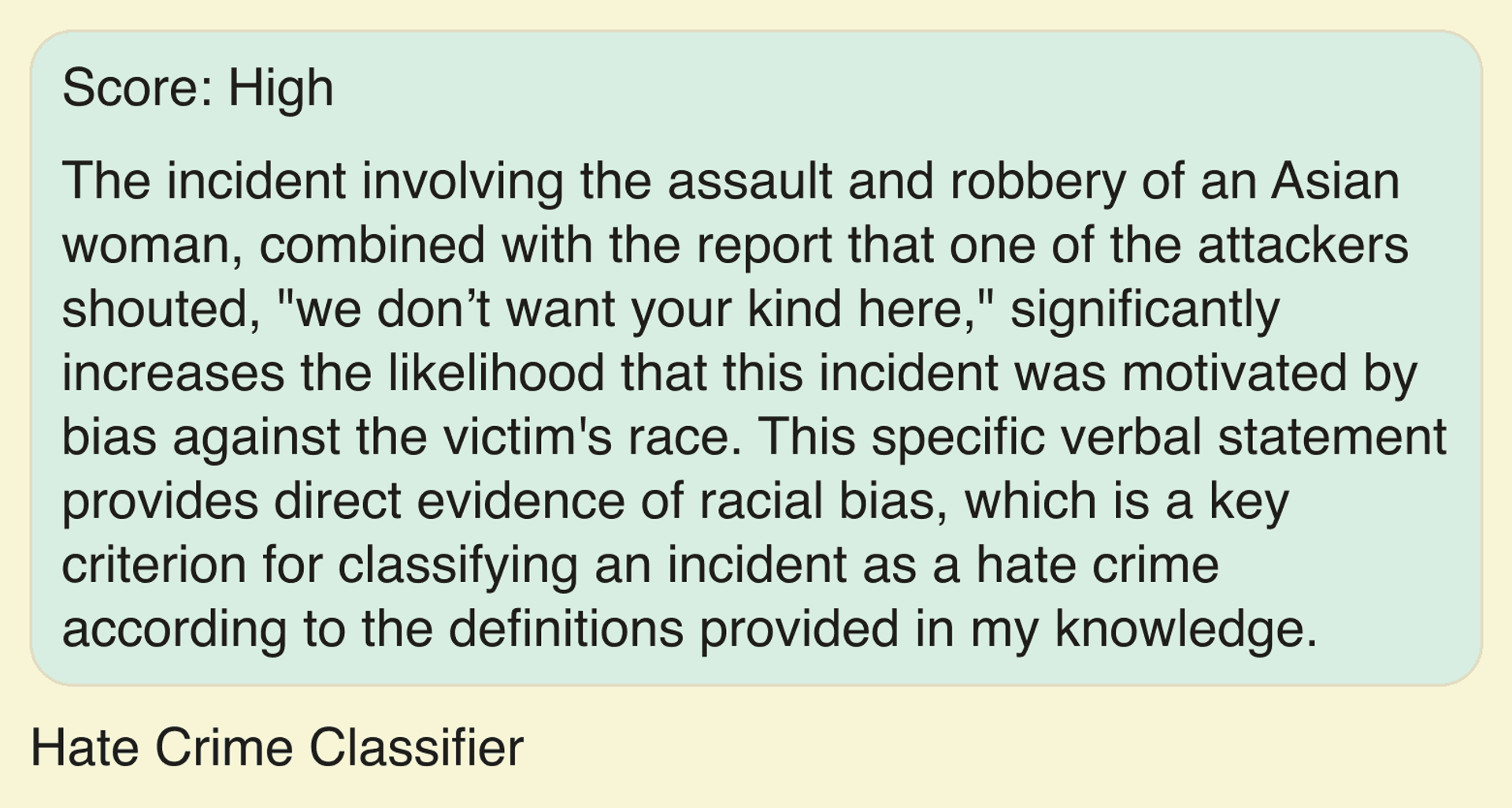
Next I changed the victim to a woman wearing a MAGA cap, and said the attackers had been seen coming from an anti-Trump rally. This was the result:

In other words, bias may have prompted the attack, but that’s not necessarily a hate crime as defined by the DOJ.
The next example had as the victim an elderly lady returning from synagogue, and the attackers a group of people who had been seen tearing down posters of Israeli hostages held in Gaza.
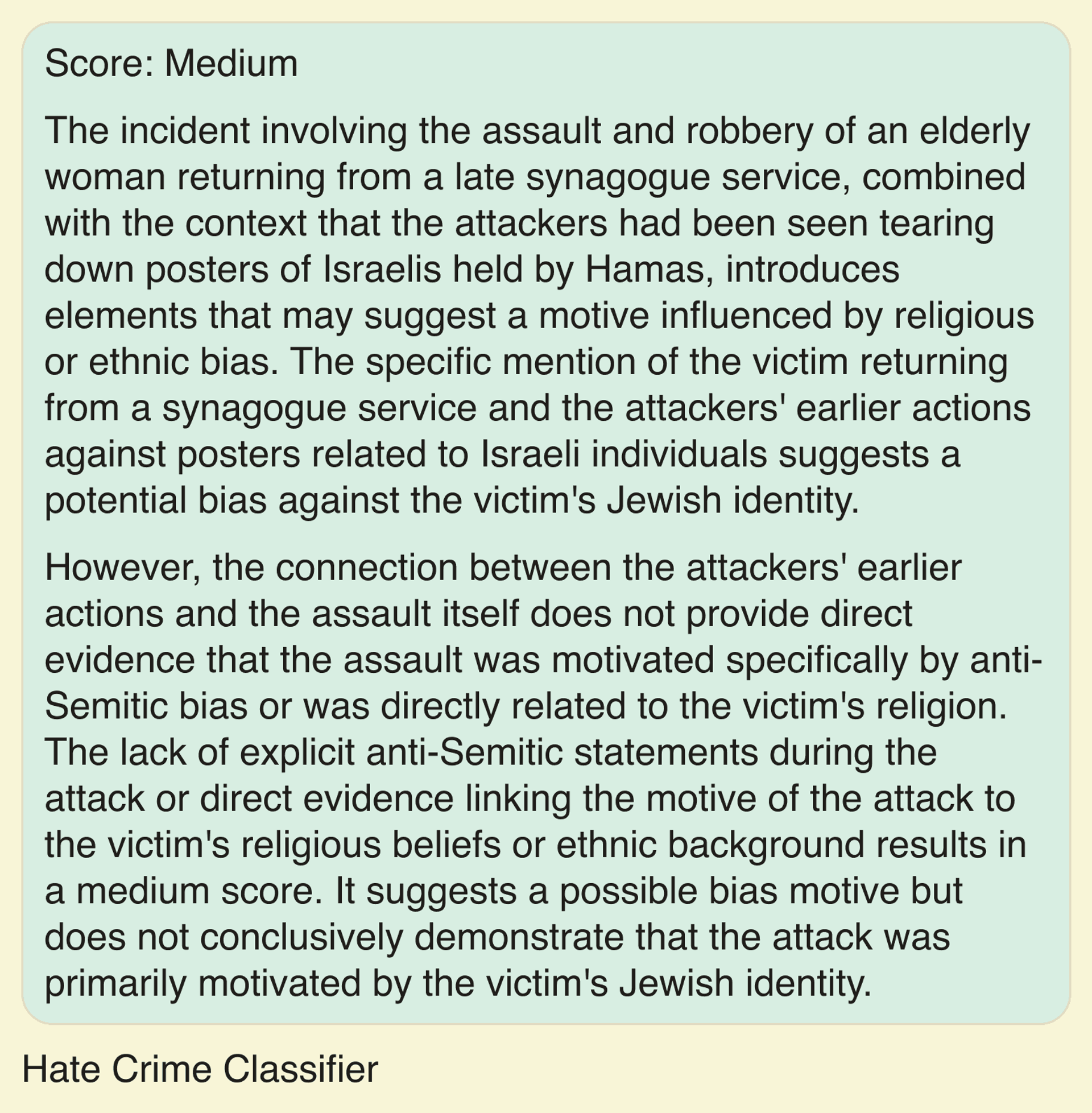
When I took out the reference to a synagogue service and made the victim a Black woman, the score changed again:
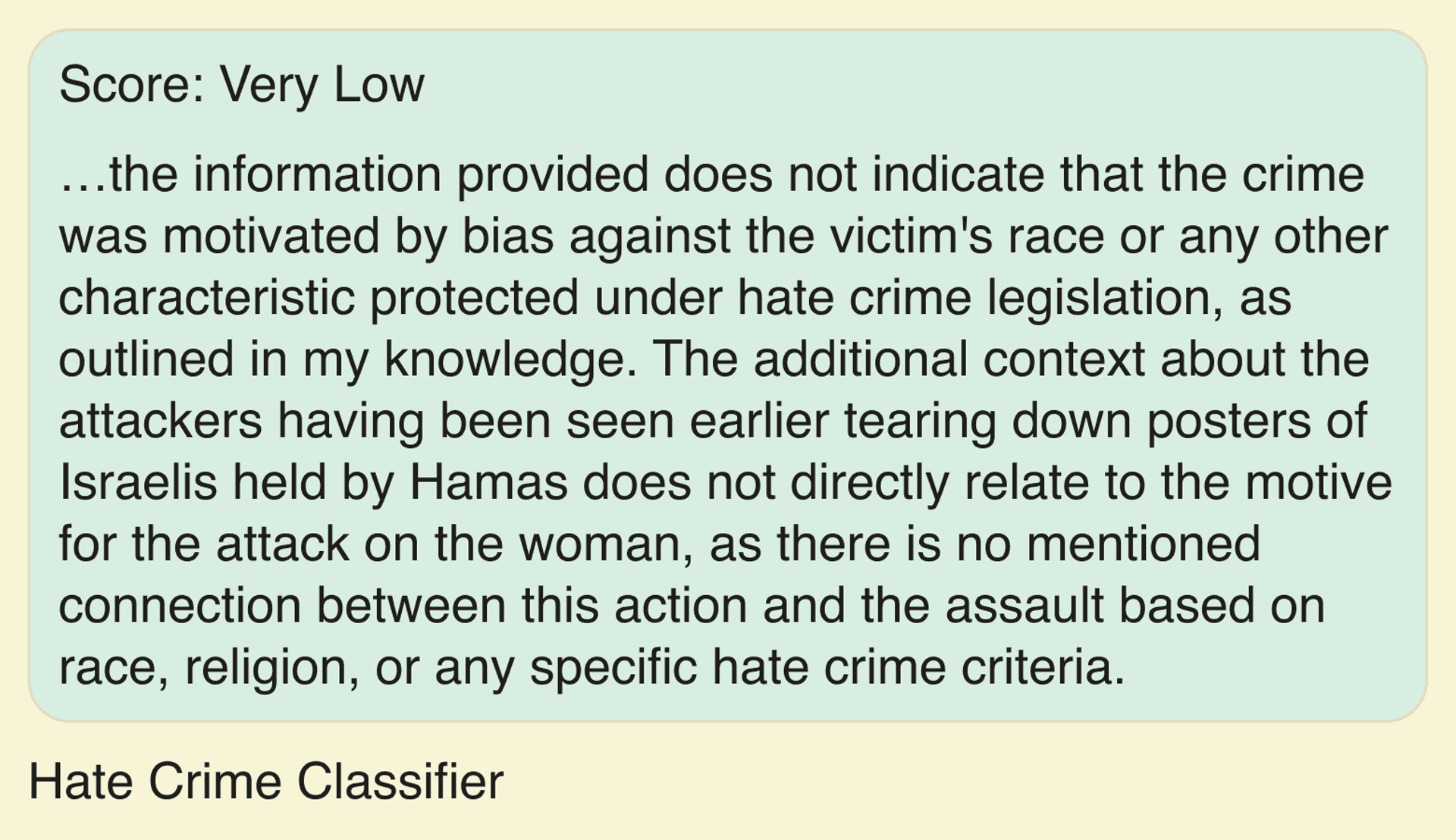

Gina’s view
This sounds like a neat parlor trick, but it’s a capability that opens a lot of possibilities.
Categorizing and classifying large amounts of information is a classic journalism — and research — problem. And sometimes that’s a relatively easy job — was this person convicted of a crime or not? Did that patient recover after the treatment or not? And sometimes it’s complicated and nuanced, such as when trying to decide if a particular post violates a complex set of community standards. That requires a level of understanding of context and language.
Machine learning — where a computer “discovers” unspoken rules and relationships — can be an incredibly valuable method for sorting this sort of in information. That’s how image recognition has progressed dramatically in recent years. But it can be prone to making unintended connections (a hiring algorithm once decided that being named “Thomas” was a factor in being successful at a particular company) and building in existing biases into its understanding of the world.
This process turns it on its head — put rules, even complex ones, into a system and ask the machine to use its facility with language to interpret whether examples fit into then or not.
My quick experiment showed there’s some real promise here. In particular, it “understood” that a woman coming from a synagogue could be Jewish, and that people tearing down posters of Israelis held in Gaza might be biased against Jews. And that, equally, those motivations might not apply to an attack on a Black woman. That’s pretty nuanced.
So I took it another step, to see how well it might handle even more complex instructions. I took the Trans Journalists Association style and coverage guidelines, as well as a few other documents on misinformation, and asked a bot to give feedback about (real) stories on the level of misinformation and misframing about trans issues in them — a much more involved exercise that required the bot to not only assess what information was in the piece, but also what context might be missing.
For example, I fed in a long piece focused on “detransition,” when trans people cease or reverse their transition. The article was mostly accurate in terms of facts, but the bot called out some contextual omissions, among them:
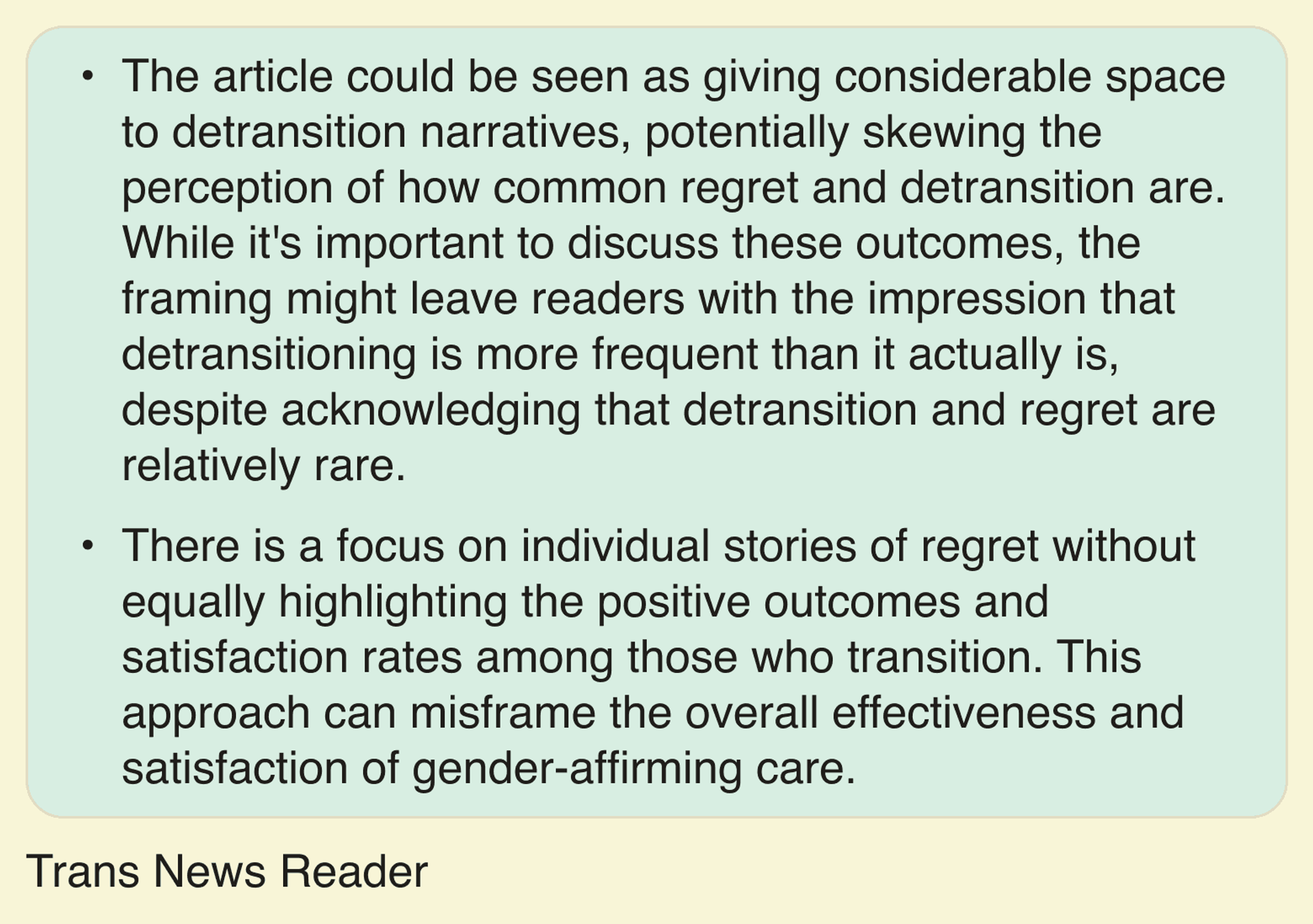
Not bad. (To be clear, you don’t have to agree with the TJA’s guidelines; the broader point is that bot knows how to read and interpret rules it’s given.)
And there are other potential use cases. Another student I know is looking at violations of New York City Housing Authority guidelines, and plans to see if a bot can accurately flag when a report identifies a potential issue, and if so, which rule it falls afoul of.
All of which to say — here’s another potential use for generative AI that focuses on a few of its key capabilities, rather than try to make it a Know Everything machine.

Room for Disagreement
These systems may actually work better and faster than human journalists at sifting through information to find patterns. But can you really ask a reader to trust you — or a policy-maker to make policy based on your findings — if you can’t explain them? Maybe we will, at some point, develop faith in the remarkably close approximations LLMs can make. But part of journalism is a contract with the audience, and I’m not sure the audience has signed on to this.
Another major issue in trying to build industrial-strength AI agents, at least off publicly available systems like OpenAI’s Plus plan, is the inconsistency of responses. The LLMs are constantly evolving in response to user inputs, and that can mean different results on different days. There are some ways around this, including setting off a series of bots on the same task and comparing the results — which is also how Mechanical Turk exercises are often also run — but it adds a level of fuzziness to any experiment.
That said, these bots aren’t meant to give definitive answers, but to help journalists sift through large amounts of information so they can focus on the subset most likely to be helpful. At the end of the day, both the practice of journalism — and audiences — will require the involvement of humans at the end of the process.

Notable
- Columbia Journalism Review published a long report on the impact of AI on journalism, concluding, among other things, that “as with any new technology entering the news, the effects of AI will neither be as dire as the doomsayers predict nor as utopian as the enthusiasts hope.”
- The Guardian profiled a 300-plus-year-old newspaper that has embraced AI tools in its newsgathering and production processes.
- Semafor recently announced the launch of a new news feed, called Signals, that uses tools from Microsoft and OpenAI to help extend the reach and breadth of its journalists.